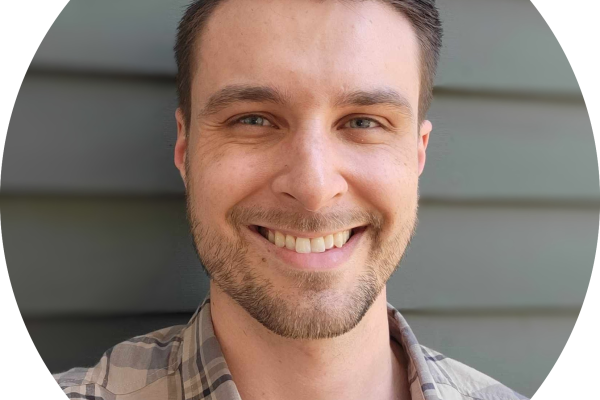
Progress and Problems in Deep Learning-Based Latent Variable Modeling
Over the past few years, researchers have made progress in understanding how deep learning methods can be used to enhance traditional latent variable models by making these models more flexible and faster to fit. However, many interesting problems have not yet been thoroughly investigated. In this talk, I’ll discuss the recent progress and demonstrate new software for fitting deep learning-based latent variable models. I’ll conclude with some preliminary work on two problems I am investigating for my dissertation: modeling (1) non-normal latent variables and (2) intensive longitudinal data.
Second Talk Details.
- Time: 3:10 PM to 4:40 PM EST
- Location: LZ 120
- Title: A Deep Learning Approach to Modeling Intensively Measured, Longitudinal, and Multidimensional Item Responses
Description: Smartphones and wearable sensors now afford social scientists unprecedented opportunities to collect intensive longitudinal data (ILD). ILD consisting of participant responses to repeated assessments taken at short intervals is useful for gaining insights into the fine-grained dynamics of experiential and behavioral processes. In this talk, I'll discuss my recent work on developing a deep learning framework for second-order latent growth modeling using ILD. Specifically, my approach combines stochastic differential equations (SDEs; a broad class of continuous-time time series models) with neural networks to produce second-order latent growth models (SLGMs) with greatly enhanced modeling capacity relative to conventional methods. In addition to more flexible SLGMs, this new framework includes models for the temporal distribution of measurement occasions and response missingness as well as statistical tests for goodness-of-fit and model selection. I will showcase some recent simulation results as well as an application to modeling emotion-switching in individuals with borderline personality disorder